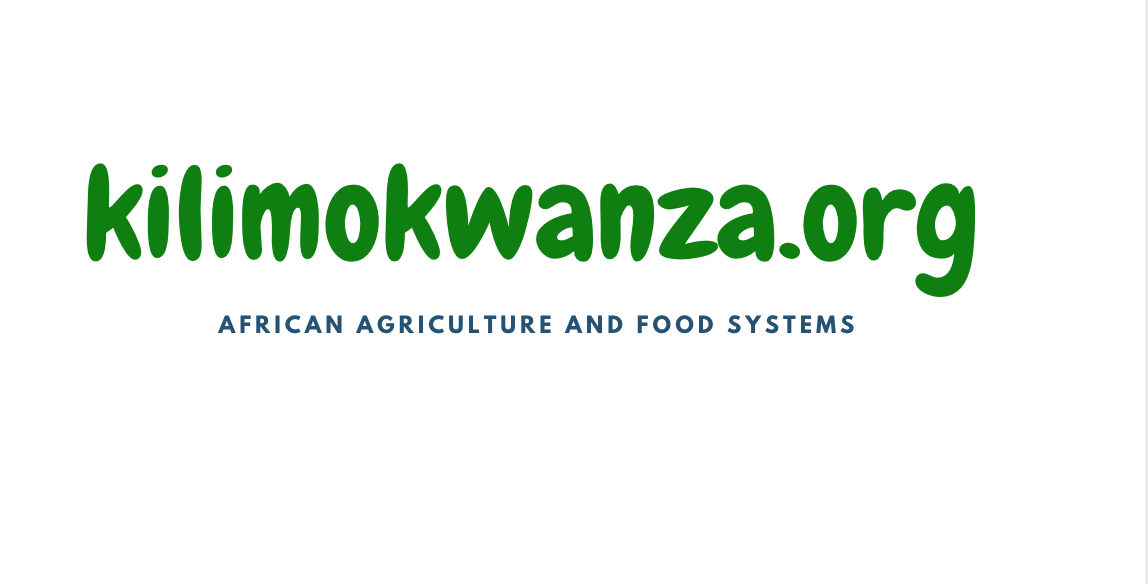
By Anthony.Muchoki@gmail.com
An international team of researchers have created an innovative, high-resolution Soil Information System for the continent of Africa. The system allows for detailed mapping of soil nutrients and properties at a 30m spatial resolution, providing invaluable data for sustainable agriculture, environmental programs, and the formulation of soil and fertilizer policies. This monumental achievement is an upgrade from previous maps, which were only available at generalized scales or in some cases, not available at all.
The research team, led by Tomislav Hengl from EnvirometriX Ltd, Netherlands, used an unprecedented number of soil samples (approximately 150,000) and Earth Observation data to predict soil properties like pH, organic carbon, and nitrogen content. They employed a two-scale 3D Ensemble Machine Learning framework, incorporating cutting-edge technologies, such as Sentinel-2 and Landsat images, for data analysis and prediction.
Accuracies of the predictions varied, with soil pH having the highest accuracy level (CCC = 0.900) and properties like extractable phosphorus (CCC = 0.654) and sulphur (CCC = 0.708) being less predictable. Covariates including Sentinel-2 bands SWIR (B11, B12), NIR (B09, B8A), and Landsat SWIR bands, alongside vertical depth derived from 30 m resolution DTM, proved to be the most influential at this resolution. Climatic data images, however, were identified as the most important variables for predicting soil chemical variables at a continental scale.
This research was made possible by the growing availability of soil samples collected by various government and NGO-funded projects. The continent-wide soil map aims to close yield gaps, guide soil and fertilizer investments, support environmental programs, and assist with targeted nutritional interventions. The database is publicly available, providing an invaluable resource for regional research and development.
The team’s efforts signify a leap forward in predictive soil mapping, incorporating machine learning, soil science, and statistical approaches. The resulting detailed data on African soil properties and nutrients promise to inform sustainable agriculture practices and policy decisions across the continent.
Utilizing an innovative two-scale ensemble machine learning framework, the researchers produced soil property and nutrient maps at an unprecedented 30-meter spatial resolution. The work encompasses various soil components, including pH levels, organic carbon, total nitrogen, carbon, and effective Cation Exchange Capacity (eCEC) and extractable elements such as phosphorus, potassium, calcium, magnesium, sulfur, sodium, iron, and zinc.
Previously, soil maps of Africa were only available at highly generalized scales, leaving many countries unmapped. The scarcity of accurate soil information has been a major impediment to agriculture and environmental policy in Africa. However, with an increase in soil samples collected at field point locations, funded by various government and NGO projects, this breakthrough now presents an opportunity for detailed continent-wide soil analysis.
The researchers applied machine learning models to a comprehensive compilation of around 150,000 soil samples and Earth Observation data. They used satellite imaging with 250-meter and 30-meter resolutions from platforms like MODIS, PROBA-V, SM2RAIN, Sentinel-2, and Landsat.
The study’s spatial Cross-Validation results showed varying levels of accuracy, with soil pH being the best-performing soil component and extractable phosphorus and sulphur showing less predictability. Sentinel-2 bands and climatic data images were found to be the most important variables for predicting soil chemical variables at a continental scale.
This new 30-meter Soil Information System has vast potential applications. It will be instrumental in guiding soil and fertilizer policies, investments, and agronomic advice to improve crop yields. It can also aid environmental programs and inform nutrition interventions.
Predictive soil mapping, a sub-field of Applied Predictive Modeling, is an interdisciplinary amalgamation of statistics, soil science, and machine learning. The consortium’s goal was to produce the most accurate and objective predictions of soil variables either for bulk estimates or specific soil depths. The predictive models were trained using terrain attributes, parent material maps, climatic and vegetation maps, and surface reflectances.
The consortium involved entities from academia, research institutes, NGOs, and the private sector across Europe, Africa, and the United States.